As he opened the courtroom door, he didn’t know his child custody hearing would involve an attack with an angry audio he never spoke, that his ex-wife created by Googling.
Deepfakes aren’t just about politicians and celebrities, they are hitting home. And they are also opening up creative avenues for spoken word, audiobooks, and so much more.
Jason Rodgers shares insights from his Masters Research, with a focus on media forensics, criminal justice deepfakes, how to create them and how to spot them.
Listen on Apple || Spotify || YouTube
Take the Deepfake Audio Test (answers - bottom of page)
00:00:00 How Deepfakes Work
00:01:09 Intro to Jason's Deepfake Forensic Audio Research Project
00:02:50 Criminal Case with Deepfake Audio Evidence
00:05:13 The Liar's Dividend - Calling it all Fake!
00:07:53 Key Indicators of a Deepfake
00:10:49 Jason's Listening Experiment - Spot the Fake!
00:19:13 Actual Deepfake: Martin Lewis UK Celeb Fake
00:22:22 Tell a Deepfake from a Real One
00:24:44 Watermarking Audio - Protecting against Deepfakes
Jason Rodgers is a Producer — Audio Production Specialist with Bitesize Bio
Affiliation: Liverpool John Moores University (LJMU), Applied Forensic Technology Research Group (AFTR)
Qualifications: BSc Audio Engineering - University of the Highlands & Islands (2017), MSc Audio Forensics & Restoration - Liverpool John Moores University (2023)
Professional Bodies: Audio Engineering Society - Member, Chartered Society of Forensic Sciences - Associate Member (ACSFS)
LinkedIn: https://www.linkedin.com/in/ca33ag3/
Jason is currently writing a paper around the challenges to credibility and qualifying factors for forensic audio professionals acting as expert witnesses in court due to the lack of standards relating to audio analysis. This is in part to identify issues for follow-up research into developing a framework for forensically authenticating audio files (especially those suspected of being deepfake).
The AI Optimist Episode #22 Summary
We explore Jason Rodgers' master's research into deepfake audios and deepfake technologies in video, their impact on criminal justice, historical visual deepfakes, and an audio listening experiment for authenticity detection.
Introduction
· Overview of the podcast episode featuring an interview with Jason Rodgers about his master's research on evaluating authenticity thresholds in deep fake audio and implications for criminal justice
· Rodgers' origin story in deepfakes and their use in criminal cases after learning media forensics teams aren't looking for them.
· His research focused on changing how we present audio evidence to identify fakes.
· From Jason Rodgers' Masters research:
"At the time of writing, there have been a handful of instances where deepfakes have made their way into the courtroom.
One example that has been written about concerned a child custody hearing. As the case involves children the proceedings are protected, however, the reported media writes that the mother's party had created deepfake audio which depicted the father as aggressive and threatening and submitted it to the court as evidence (AIAAIC - Dubai deepfake court evidence, 2023).
Experts later determined that this audio was not legitimate by examining the metadata of the audio file, but in today's world, it is very easy to manipulate or even erase metadata."
Deepfakes in Criminal Cases
· An example court case discussed where a mother used deepfake audio to paint the father as abusive in a child custody case.
· The fake audio was presented as evidence but found to be AI-generated through metadata traces.
· We explore the forensic community's struggle to adapt to rapid technological changes.
· Jason shares the difficulty in proving Authenticity in court due to proprietary detection tools.
· This custody case shows the potential for deepfakes to influence legal claims improperly.
"A 'deepfake' audio recording was used in a UK child custody battle in an effort to discredit a Dubai resident, it has been revealed.
Byron James, a lawyer in the emirate, said a heavily doctored recording of his client had been presented in court as evidence in a family dispute.
In the edited version of the audio, the child's father was heard making direct and "violent" threats towards his wife.
But when experts examined the recording, they found it had been manipulated to include words not used by the client.
"This is a case where the mother has denied the father access to the children and said he was dangerous because of his violent and threatening behaviour," Mr. James said.
The mother used software and online tutorials to put together a plausible audio file.
"She produced an audio file that she said proved he had explicitly threatened her.
"We were able to see it had been edited after the original phone call took place, and we were also able to establish which parts of it had been edited.
"The mother used software and online tutorials to put together a plausible audio file."
How Deepfakes Work
· Early deepfakes had detectable flaws like lack of blinking, but creators identified and fixed this over time.
· Generated ear lobes used to be an indicator, but improved generative AI fixes this.
· As soon as academic research identifies a way to detect fakes, creators upgrade the tech.
· Reliable indicators are increasingly complex to find.
Adapting Forensic Analysis
· AI detection tools carry limitations and proprietary secrets, so manual authentication frameworks are needed.
· Rodgers is focusing on developing a methodology for audio forensics.
· Experts view audio on multiple levels, fidelity, metadata, cadence, pronunciation, etc., to identify possible manipulation.
The "Liar's Dividend"
· Deepfakes make the public distrustful of media authenticity and allow people to dismiss objective evidence as fake.
· Politicians deny accurate negative coverage as deepfakes.
· Verifying actual footage strengthens distrust since quality fakes are possible.
From Boston University's Research:
"Deep fakes will prove useful in escaping the truth in another equally pernicious way. Ironically, liars aiming to dodge responsibility for their real words and actions will become more credible as the public becomes more educated about the threats posed by deep fakes.
Imagine a situation in which an accusation is supported by genuine video or audio evidence. As the public becomes more aware of the idea that video and audio can be convincingly faked, some will try to escape accountability for their actions by denouncing authentic video and audio as deep fakes.
Put simply, a skeptical public will be primed to doubt the Authenticity of real audio and video evidence. This skepticism can be invoked just as well against authentic as against adulterated content. "
Take the Deepfake Audio Test (answers - bottom of page)
Rodgers' Research Methodology
· He creates a 10-question audio quiz with three samples per question, some real and some AI-generated.
· Testing the quiz with the public and specialists like audio engineers and media forensics teams.
· Some personal bias arises, even among experts familiar with the voices.
· Background noise samples simulate real-world conditions:
· The experiment involves identifying real vs. fake audio clips.
· Various scenarios (e.g., telephone filter, street noise) are tested.
· Challenges in differentiating authentic audio due to improving deepfake quality.
· It understands the importance of considering the pros and cons of the audio content instead of making definitive judgments about real vs. fake.
Live Test of Rodgers' Samples
· Dunn takes the quiz and shares the thought process in determining real vs fake.
· Dunn is wrong on the first round, even with a lack of personal familiarity with the speaker (Scottish accent).
· Dunn demonstrates bias in the second experiment test, where a familiar voice sounds authentic.
· Takeaways:
o Bias affects specialists' evaluations.
o It's easier to fake an unfamiliar voice.
o Presentation quality and clarity influence perceptions of Authenticity.
Deepfake Video Examples
· An example of a high-quality fake video impersonating famous UK consumer advocate Martin Lewis.
· Detecting well-made fakes is complex and requires scrutinizing facial details.
· Evaluating fluid movements like turning the head and facial expressions is harder.
Challenges Judging Authenticity
· With good-quality video and audio editing tools, altered media is commonplace.
· Changes how we establish a baseline of what's "real."
· There are multiple factors to analyze, like fidelity, metadata, vocal patterns, etc.
· Watermarking proposals to embed source info during content creation.
o The Coalition for Content Provenance and Authenticity (C2PA) addresses the prevalence of misleading information online.
o Manual and AI-based methods for detecting fakes are limited.
o There's a need for complete analysis beyond metadata and visible indicators.
Conclusion
· Deepfakes represent a significant challenge as technology improves.
· Diligence in verifying Authenticity before sharing or acting on media is now a requirement.
· Holistic evaluation combining technological solutions and trained human judgment is needed.
How to Spot Deepfakes – a Simple Action Plan:
· Scrutinize media, especially high-stakes info related to individuals.
· Look for odd visual artifacts and inconsistencies in facial features/expressions.
· Listen for unnatural speech patterns and vocal anomalies.
· Cross-reference sources, when possible, to verify.
· Consider adding watermarks during content production.
· Support efforts to responsibly advance detection technology.
· Remain skeptical but avoid knee-jerk "fake news" reactions without evidence.
· Be cautious about assuming Authenticity in digital content.
· Learn to identify potential deepfake indicators.
· Stay informed about the evolving nature of deepfake technologies.
What Are Deepfakes and How Are They Created?
· Deepfakes blend the words "deep learning" and "fake." They utilize AI and machine learning algorithms to manipulate or generate visual and audio content with a high potential to deceive.
· The creation process generally involves two AI algorithms: one creating the content (a "generator") and another detecting its artificial nature (a "discriminator"). This adversarial process, known as Generative Adversarial Networks (GANs), refines the output to be realistic.
· Deepfakes refer to media (images, audio, video) manipulated and falsified using artificial intelligence and machine learning techniques.
· The name is "deepfakes" because they utilize deep learning, a complex form of neural network modeling that analyzes and reconstructs intricate patterns such as those found in human speech and imagery.
Deepfakes represent a challenge in the digital age, blurring the lines between reality and fabrication. As technology advances, the ability to create convincing deepfakes becomes accessible, increasing the risks of misinformation and exploitation.
Solving the problem demands a combined effort in technology development for detection, legal frameworks for regulation, and public education to foster media literacy.
Discerning Authenticity will be a technological challenge and a fundamental skill for participating in a digital society.
Tools Used:
· Popular Tools: DeepFaceLab, FaceSwap, Descript, ElevenLabs, Speechify, and Adobe After Effects create deepfakes. More recently, AI models like GPT-4 and others developed by companies like DeepMind and OpenAI are advancing.
· Accessibility: The accessibility of deepfake technology is increasing, with apps and software becoming user-friendly for non-experts.
Challenges to social media:
· Content Moderation: Social media platforms need help keeping up with detecting and moderating deepfake content.
· Awareness: Users must be critical of their media, understanding that seeing is no longer believing.
Diving Deep into Deepfake Technology
Specifically, deepfake creation relies on generative adversarial networks (GANs). Design involves training two AI models against each other - one generates synthetic media, and the other serves as a detector scrutinizing the output authenticity.
These adversarial dynamic pressures both models to evolve and become highly adept at their role.
The generative model uses large volumes of source media data like photos or videos of an individual to learn their facial expressions, speech patterns, and other visual/vocal details.
The detector model then reviews the outputs, flagging areas that don't seem genuine. The generator integrates this feedback to refine its fakes while learning to thwart the detector.
After extensive iterations, the generator becomes skilled enough to produce highly lifelike fabrications that fool AI and human observers.
The fully trained model is applied to synthesize new video or audio of the target individual appearing to say or do things they never actually did.
Deepfake programs like Zao, Avatarify, and Reface leverage these techniques to generate videos of a source individual, like a celebrity, synchronized to another person's facial expressions.
Advanced platforms allow manual editing of faked imagery and audio or generating new videos from text prompts.
Use Cases and Examples
· Politics: Altered videos of politicians, like one of Nancy Pelosi, slowed down to make her appear drunk to make headlines.
· Entertainment: Deepfakes are being used in film and television to de-age actors or bring deceased stars back to life, like Peter Cushing in "Star Wars."
· Misinformation: Deepfakes appear in misinformation campaigns, impacting public opinion and potentially election outcomes.
While deepfake technology holds exciting promise for entertainment, education, and other fields, it also poses significant risks for misuse.
The most infamous applications involve non-consensual use to create "revenge porn" or fake news/disinformation.
Deepfakes help offenders carry out fraud and harassment—criminals using AI-generated audio of a CEO's voice to trick an employee into transferring $243,000.
Fake celebrity pornographic videos harass and exploit without consent. Victims describe the experience as a form of assault.
However, deepfakes also bring positive applications. Filmmakers use them to restore historic speeches by figures like Harriet Tubman or create updated scenes featuring a young Princess Leia in Rogue One.
Visual effects artists utilize AI tools to build realistic digital doubles. Avatarify and similar apps allow comedic face-swapping for entertainment.
Educational deepfakes help illustrative concepts - an AI replica of Richard Feynman delivers lectures on physics and drums up renewed interest.
AI voice duplication enables podcasters and creatives to reuse vocals from historical speeches or literature without costly voice actors. The tech also expands accessibility for those unable to speak or record their voices.
Societal and Ethical Challenges
Usage:
· Fooling and Deceiving: Initial deepfakes gain notoriety for their use in creating fake pornography and revenge porn, exploiting celebrities and ordinary people. They've also created false narratives and misinformation.
· Informing About Challenges: Awareness campaigns and educational content use deepfakes to demonstrate their potential harm and encourage critical media consumption.
Challenges to Society:
· Impact on Trust: Deepfakes erode trust in media, making it difficult for people to discern what's real or fake.
· Legal and Ethical Concerns: They raise questions about consent, privacy, and the ethical use of someone's likeness.
· Security Threats: Deepfakes pose security threats, including the potential for false evidence in legal cases or manipulating stock markets with fake news
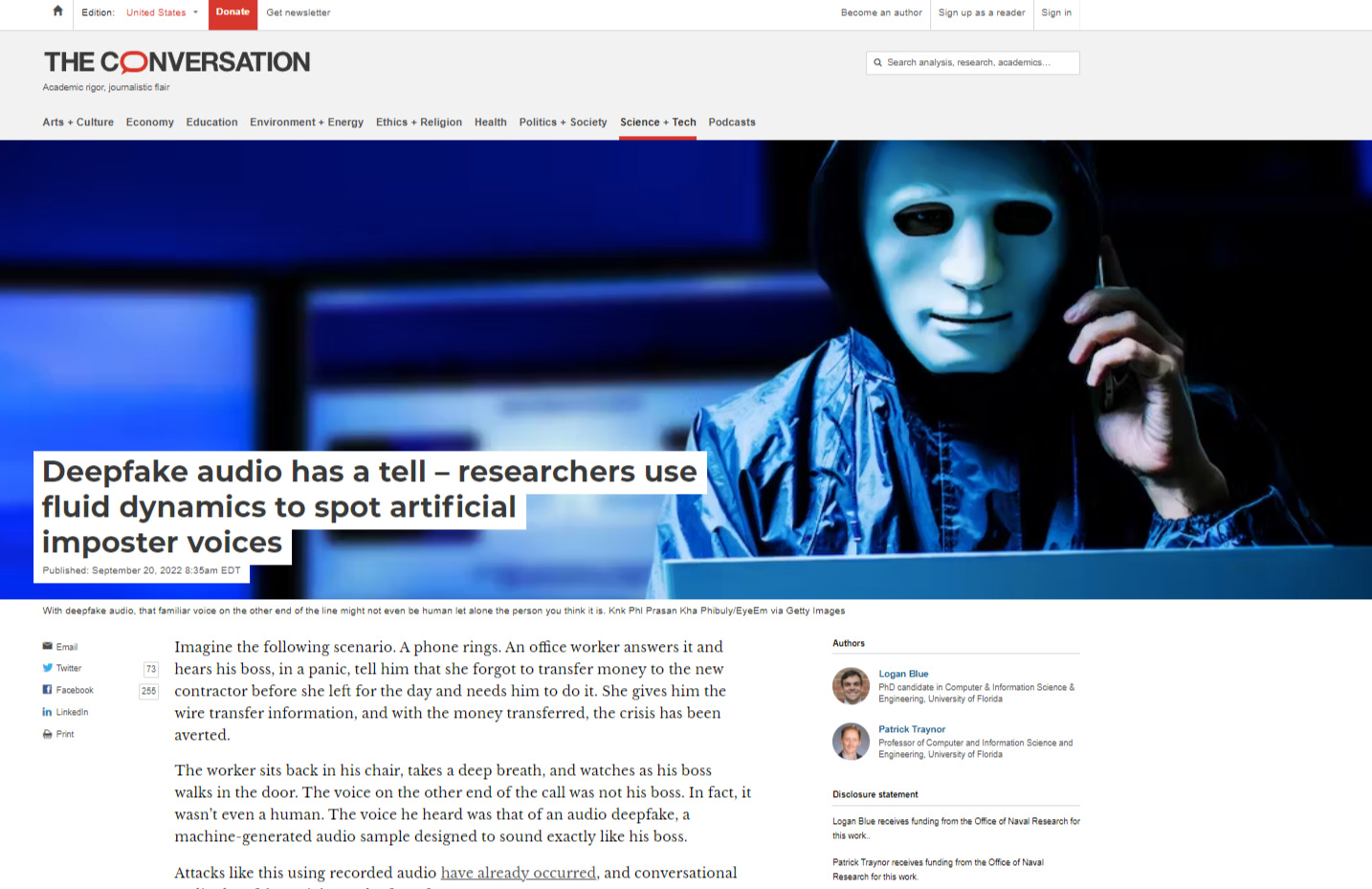
.Importance of Deepfakes:
· Highlight the need for public awareness and technological literacy.
· Emphasize the urgency for improved media forensic methods.
The unchecked proliferation of deep fakes poses threats on several fronts:
Trust & Misinformation - Widespread synthetic media weakens public confidence in online content and images – even real ones get discounted as "fake."
The lack of confidence benefits bad actors who easily dismiss genuine negative coverage as deepfakes. Verifying real clips heightens distrust.
Politics & Civic Discourse - Manipulated or entirely fabricated video/audio of candidates circulated near elections impacts results while avoiding consequences of defamation. Even releasing real clips out of context damages reputations.
Psychological Harm - Seeing oneself in traumatic deepfake situations negatively impacts mental health and leads to self-harm in severe cases. The possibility also engenders constant anxiety about victimization.
Discrimination & Exploitation - Certain vulnerable groups face a disproportionate risk of deepfake victimization, especially non-consensual pornographic use. The threat further marginalizes them psychologically and socially.
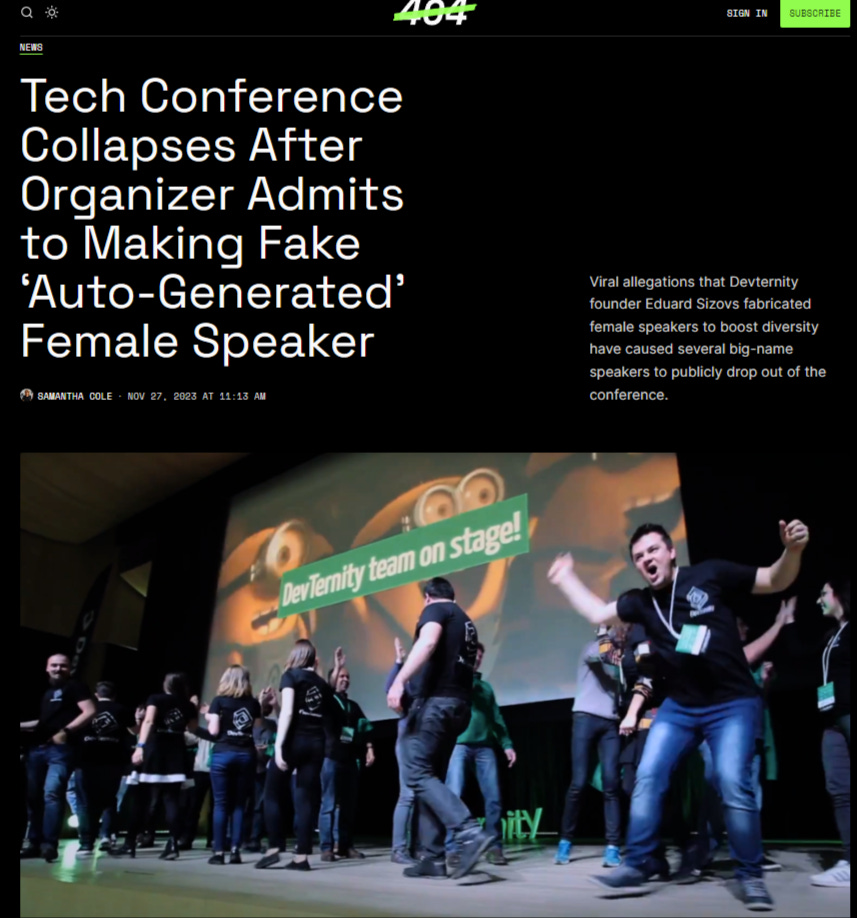
To address these risks, experts recommend four essential precautions:
1. Critical Thinking - Scrutinize unverified viral media and verify using trusted, impartial sources before circulating or reacting.
2. Consent & Privacy - Before creating or sharing media showing others, obtain explicit consent and consider long-term unintended circulation and misuse.
3. Verification Standards - Develop institutional protocols requiring rigorous AI and manual examination of media authenticity before acceptance and publication.
4. Attribution Technology - Embed verifiable digital attribution details during media recording to provide trusted source history, easing forgery detection.
Responsible governance of deepfake technology, balancing innovation with ethics, is crucial to managing the dangers unchecked development poses.
Like media literacy helps protect against misinformation, a critical eye aware of manipulation markers like visual artifacts aids self-defense.
We must evolve societal resilience alongside AI to ensure a just future.
Deepfakes represent a significant challenge in the digital age, blurring the lines between reality and fabrication. As technology advances, the ability to create convincing deepfakes becomes more accessible, heightening the risks of misinformation and exploitation.
The new reality necessitates a combined effort in technology development for detection, legal frameworks for regulation, and public education to foster media literacy.
As we progress, recognizing the Authenticity of digital content will be a technological challenge and a fundamental skill for participating in a digital society.
Share this post