Swimming in the a priori AI bias sea 🏊 and AI Copyrights -
with flashes of what's possible, like from the New England Journal of Medicine on LLM usage:
(what's your take - could this approach apply to copyrights?)
Scientific research and writing using LLMs - does that make sense, as explained below?
Listen on Apple || Spotify || YouTube
00:00:00 AI Copyrights new Twists
00:00:59 NY Times Lawsuit
00:01:49 Where we are - poor usage and so so data
00:05:56 New AI Copyrights - what's involved
00:08:05 NEJM AI accepts GenAI Science Research
00:10:45 Sports Illustrated and CNET Hide usage
00:11:27 The First P of AI Copyrights - Pliable
00:14:46 2nd P - Permission
00:15:40 3rd P - Protection
00:18:15 Hollywood Strike that will use AI
00:19:52 4th P - Precedence
00:21:17 Generative AI Copyrights Summary
"At NEJM AI, we have therefore elected to allow the use of LLMs.
Our two key conditions are
1️⃣ First, that the use of LLMs is appropriately acknowledged by the authors.
This standard is the same as for any tool or resource that is used in a substantive way by authors in their scientific work, including experimental reagents, animal models, data sets, software systems, or third-party copyediting services.
2️⃣ Second, we require that the authors be completely accountable for the correctness and originality of the submitted work.
Likewise, the same quality standards for clarity, exposition, and strength of the scientifi arguments will be applied to all papers submitted to NEJM AI, regardless of how the text was generated.
Using an LLM does not absolve one of the responsibility to write well and to avoid plagiarism."
Exploring the boundaries and horizons of GenAI - people will be creating images and written works with GenAI.
Existing copyrights demand that a human create/originate the idea.
In 2 earlier pods, we look at a few relevant cases.
This week, we dive into how copyright might look with AI involved from 4 current use case studies.
What's your view?
Should scientific research and writing be allowed to use LLM's, similar to the model the New England Journal of Medicine outlines?
Episode 24 - AI Copyright Resistance is Futile
Outline
I. Introduction to AI Copyrights
- Definition and current status
- Importance in upcoming years
II. The 4 P's Framework of AI Copyrights
- Pliable
- Permission
- Protection
- Precedence
III. Use Case Studies
- New York Times Lawsuit
- New England Journal of Medicine
- Intel's MAMC
- Hollywood Writer's Strike
IV. Copyrights for Humans Only?
- Central issues and debates
- Suggestions for responsible usage of generative AI
V. Conclusion and Action Plan
- Key takeaways
- Recommendations for managing AI copyrights in the future
Introduction to AI Copyrights
AI copyrights refer to the intellectual property protections granted to creations generated by artificial intelligence systems. As generative AI becomes more advanced and widespread, there are open questions about whether and how copyright law applies.
Key issues include whether AI-generated works qualify for copyright and who owns the rights - the AI system developer, the prompt engineer, or someone else.
Intellectual Property Rights: It respects artists' intellectual property by preventing unauthorized replication of their work but may raise questions about the extent of these rights in the digital realm.
Fair Use and Innovation: A balance between protecting content and allowing for fair use is crucial for innovation and creative freedom in AI development.
Privacy and Consent: Ensuring that AI does not misuse personal data, especially in generating content, is paramount.
Accessibility and Inclusivity: Such protections should not limit the accessibility of AI technology for educational, research, or creative purposes.
Legal Precedence: As AI evolves, legal frameworks must adapt, ensuring that new technologies abide by existing laws and ethical standards.
These questions have significant implications in the years ahead as generative AI transforms industries involving writing, art, music, and more.
Lawsuits and debates on this topic are already emerging, evidencing the growing importance of establishing clear AI copyright rules and protections.
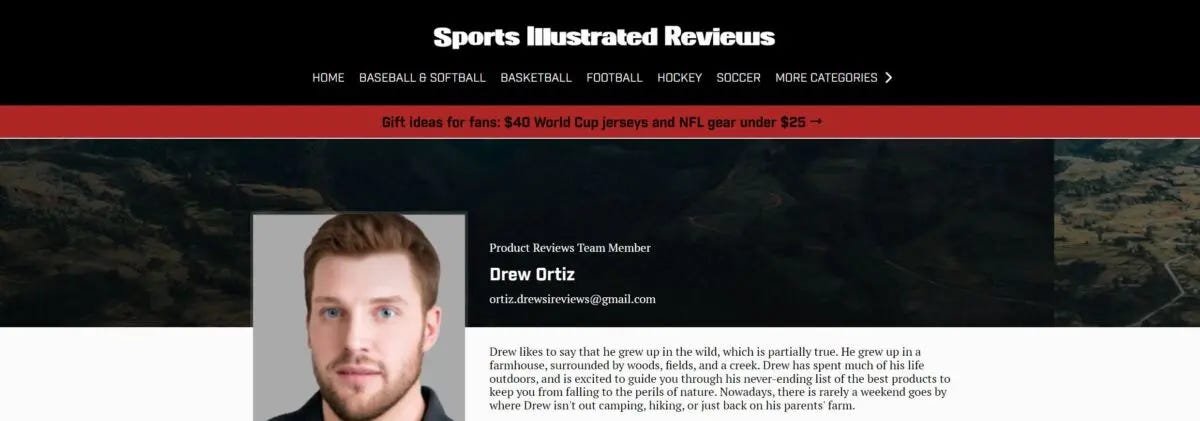
The 4 P's Framework of AI Copyrights
4 case studies look at four key dimensions shaping the reform of AI copyright law:
1. Pliable - Being adaptable to generative AI rather than rigidly opposing it. The New England Journal of Medicine provides a model where transparency and responsibility standards allow AI to augment scientific writing.
2. Permission - Requiring licensing deals and consent to use copyrighted source materials in AI training datasets, rather than scraping data without approval, as many systems have done.
3. Protection - Developing technical solutions like digital watermarking and adversarial outputs that protect artists by preventing unauthorized AI reuse of their creations. Intel's MAMC system showcases early efforts on this front.
4. Precedence - There is a need for court rulings and legal precedents to establish case law on AI copyrights, which will likely involve lawsuits progressing to the Supreme Court level.
Use Case Studies
Declan analyzes several timely use cases highlighting open AI copyright questions:
- New York Times Lawsuit - The NYT is suing OpenAI for copyright infringement over its AI model quoting NYT articles without permission. This suit speaks to licensing issues with web-scraped training data.
- New England Journal of Medicine - This scientific journal has taken a proactive stance, allowing responsible AI augmentation of health research writing following transparency and author accountability standards.
- Intel's MAMC - This AI technique distorts images to confuse generative systems, providing artists and photographers a technical means to protect visual works from unauthorized AI reuse.
The paper "My Art My Choice: Adversarial Protection Against Unruly AI" focuses on a method for protecting copyrighted images from being exploited by generative AI models, particularly diffusion models.
The authors present a system called "My Art My Choice" (MAMC), which allows artists to create slightly perturbed versions of their images.
These uneasy images, while visually similar to the originals, are designed to "break" diffusion models, preventing them from replicating the style or content of the protected images effectively.
Key Points:
MAMC allows artists to control the extent of perturbation in their images, balancing between image fidelity and protection.
The system uses a UNet-based architecture to create these adversarially protected images.
Experiments conducted on various datasets demonstrate that MAMC can effectively degrade the output of diffusion models when using protected images.
Critical Learnings for AI Going Forward:
MAMC highlights the importance of respecting copyright in AI, showing a practical approach to protect artists' rights.
The balance factor in MAMC provides insight into user-controlled AI systems, where users can directly influence the outcome based on their needs.
This work emphasizes the need for ongoing research into adversarial methods in AI, not just for security purposes but also for ethical and legal considerations.
For a detailed understanding, you can access the full paper here.
- Hollywood Writer's Strike - Contract debates highlight tensions between compensating human script writers and studios' desires to utilize generative writing AI for existing film franchises. Ongoing disputes illustrate challenges.
Copyrights for Humans Only?
Central issues debated include whether copyright law should remain human-centric or expand to cover AI systems, incentives around transparency when current laws reward hiding Generation AI's involvement, and ethical questions surrounding proprietary data usage and creator compensation.
While generative models produce some low-quality outputs today, their rapid advances demands proactively addressing questions surrounding consent, protection, and fair attribution.
There are reasonable arguments on multiple sides, with the need for judicious analysis and policymaking.
Suggestions for responsible usage include:
Showing care when leveraging third-party data in systems.
Indicating when outputs involve generative models to mitigate deception risks.
Exploring compensation structures that account for human creativity displaced by advanced AI.
Conclusion and Action Plan
AI copyright remains a complex terrain requiring well-informed legal rulings and responsible development policies balancing many stakeholders' interests.
It’s clear we’ll be anticipating several pending lawsuits reaching higher courts, moving toward licensing and permission frameworks rather than data scraping without approval, recognizing the need for enhanced technical protections around AI misuse and misattribution, and showing restraint around proprietary data usage and creator compensation.
Suggested action steps include:
Closely tracking emerging legal precedents on AI copyrights.
Enacting data usage review processes focused on consent and attribution ethics.
Indicating where generative models assisted creative works.
Supporting efforts to provide attribution and reasonable compensation to human creators impacted by disruptive AI systems over time.
More open questions than definitive answers. Still, careful engagement with these issues today help guide responsible AI copyright policies.
Generative AI faces significant legal challenges primarily revolving around intellectual property rights and privacy concerns.
As AI technologies increasingly interact with human-generated content, the lines of copyright infringement and data misuse become blurred.
One notable case is the New York Times lawsuit, highlighting the need for clear licensing agreements in AI-generated content.
Another solution comes from the New England Journal of Medicine (NEJM), which has implemented an AI permissions framework.
This framework provides clear guidelines on science and research with the help of AI, setting a precedent for other publishers and content creators to follow.
The Intel MAMC model offers a unique approach. It empowers content creators to protect their work from unauthorized AI replication through a permission-based system.
This model respects intellectual property rights and encourages collaboration between AI developers and content creators.
Addressing the legal challenges in generative AI requires a multifaceted approach.
Establish clear licensing agreements, develop permission-based models like the Intel MAMC, and create guidelines similar to the NEJM's AI permissions.
These solutions aim to balance the innovative potential of AI with the need to respect and protect intellectual property and privacy rights.
AI Copyright Pods - Episodes 10 and 11
💡Part 2 - Creator or Curator? The Blurring Lines of Copyright in the Age of GenAI
📢 PART 1 - Should AI-Generated content be eligible for copyright protection?
Share this post